LlamaIndex
إصدارات Python وTypescript
إصدارات Python أكثر وثيقة ، TS أسوأ؟
البداية
إنشاء بيئة
conda create --name llamaindex python=3.9.19
conda activate llamaindex
** إعداد بيئة Conda في VSCode **
Python: Select Interpreter
مكتبة التثبيت
pip install llama-index pypdf sentence_transformers
إعداد OpenAI
vim ~/.bashrc
إضافة متغير بيئي
export OPENAI_API_KEY="sk-xxxx"
التحقق
echo $OPENAI_API_KEY
** إمكانية الوصول**
تكوين في سطر الأوامر: goproxy
بداية سريعة
import os.path
from llama_index import (
VectorStoreIndex,
SimpleDirectoryReader,
StorageContext,
load_index_from_storage,
)
import logging
import sys
logging.basicConfig(stream=sys.stdout, level=logging.DEBUG)
logging.getLogger().addHandler(logging.StreamHandler(stream=sys.stdout))
# check if storage already exists
PERSIST_DIR = "./storage"
if not os.path.exists(PERSIST_DIR):
# load the documents and create the index
documents = SimpleDirectoryReader("data").load_data()
index = VectorStoreIndex.from_documents(documents)
# store it for later
index.storage_context.persist(persist_dir=PERSIST_DIR)
else:
# load the existing index
storage_context = StorageContext.from_defaults(persist_dir=PERSIST_DIR)
index = load_index_from_storage(storage_context)
# either way we can now query the index
query_engine = index.as_query_engine()
response = query_engine.query("What did the author do growing up?")
print(response)
** طريقة الإكمال المستخدمة **
/chat/completions
** البارامترات المطلوبة**
{
"messages": [
{
"role": "system",
"content": "You are an expert Q&A system that is trusted around the world.\nAlways answer the query using the provided context information, and not prior knowledge.\nSome rules to follow:\n1. Never directly reference the given context in your answer.\n2. Avoid statements like \"Based on the context, ...\" or \"The context information ...\" or anything along those lines."
},
{
"role": "user",
"content": "xxx"
}
],
"model": "gpt-3.5-turbo",
"stream": false,
"temperature": 0.1
}
** نظام موجه **
You are an expert Q&A system that is trusted around the world.
Always answer the query using the provided context information, and not prior knowledge.
Some rules to follow:
1. Never directly reference the given context in your answer.
2. Avoid statements like "Based on the context, ..." or "The context information ..." or anything along those lines.
أنت نظام أسئلة وأجوبة خبير موثوق به في جميع أنحاء العالم.عند الإجابة على الأسئلة ، استخدم دائمًا المعلومات الأساسية المقدمة وليس المعرفة السابقة.بعض القواعد التي يتعين اتباعها:
1.لا تشير أبدًا إلى معلومات خلفية معطاة مباشرة في الإجابة. 2.تجنب استخدام عبارة "وفقاً للمعلومات الأساسية"، أو "تشير المعلومات الأساسية إلى"، أو أي عبارة مماثلة.
** موجه المستخدم **
Context information is below.
---------------------
file_path: data/paul_graham_essay.txt
xxx
---------------------
Given the context information and not prior knowledge, answer the query.
Query: What did the author do growing up?
Answer:
سيناريوهات التطبيق
应用场 | 说明 |
---|---|
Q&A | 最重要 |
Chatbots | |
Agents | 高级 |
Structured Data Extraction | 有用,整理聊天记录等 |
Multi-modal |
الأساسيات
العمليات الأساسية
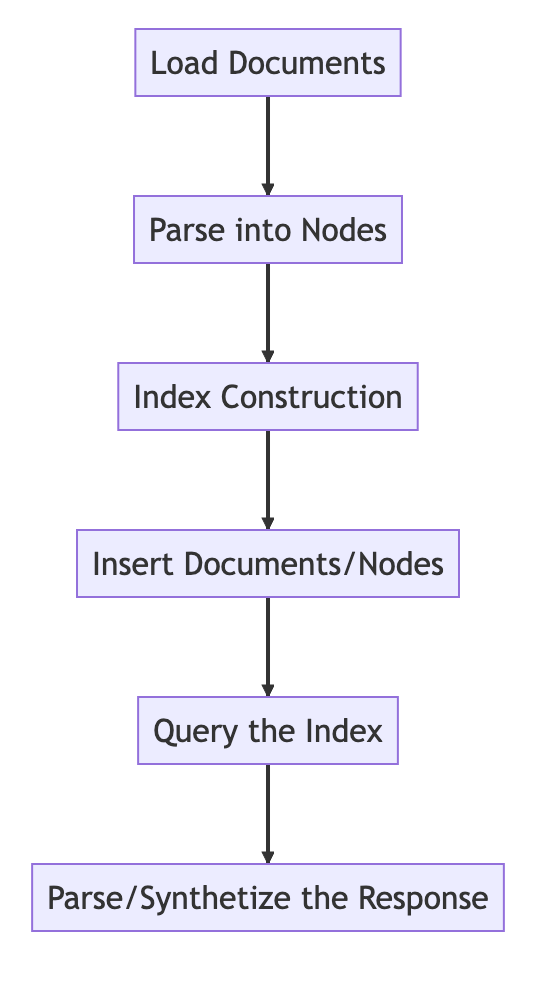
from llama_index import VectorStoreIndex, SimpleDirectoryReader
# Load in data as Document objects
documents = SimpleDirectoryReader('data').load_data()
# 切片,转成Node
# Parse Document objects into Node objects to represent chunks of data
index = VectorStoreIndex.from_documents(documents)
# Index Construction:创建索引
# Build an index over the Documents or Nodes
query_engine = index.as_query_engine()
# The response is a Response object containing the text response and source Nodes
summary = query_engine.query("What is the text about")
print("What is the data about:")
print(summary)
Chunking وNode
البيانات المصدرية --->documents --->Nodes
documents: تحتوي على معلومات النص والميتا
معرف الوثائق
document هي في الواقع فئة فرعية من Node.
"من الغريب أن يتم قطع وثيقة واحدة إلى العديد من الوثائق.
TextNode: استخدام NodeParser لتقسيم المستند إلى عقد متعددة
تضمين معرف المستند
Node > كانت هناك علاقة سابقة مع Node
1.يتلقى NodeParser قائمة بكائنات Document. 2.تقسيم نص كل وثيقة إلى جمل باستخدام تقسيم الجمل spaCy؛ 3.يتم تغليف كل جملة في كائن TextNode الذي يمثل عقدة واحدة ؛ 4.يحتوي TextNode على نص الجملة ، بالإضافة إلى البيانات الفوقية ، مثل معرف المستند ، والموقع في المستند ، إلخ ؛ 5.إرجاع قائمة من كائنات TextNode.
حفظ الوثائق والمؤشرات
كلا الطريقتين
- حفظ على القرص المحلي
- تخزين في قاعدة بيانات ناقلات
** حفظ على القرص المحلي **
import os.path
from llama_index import (
VectorStoreIndex,
SimpleDirectoryReader,
StorageContext,
load_index_from_storage,
)
import sys
# check if storage already exists
PERSIST_DIR = "./storage"
if not os.path.exists(PERSIST_DIR):
# 保存数据: Load the documents and create the index
documents = SimpleDirectoryReader("data").load_data()
index = VectorStoreIndex.from_documents(documents)
# store it for later
index.storage_context.persist(persist_dir=PERSIST_DIR)
else:
# 从磁盘加载回数据: load the existing index
storage_context = StorageContext.from_defaults(persist_dir=PERSIST_DIR)
index = load_index_from_storage(storage_context)
query_engine = index.as_query_engine()
response = query_engine.query("What did the author do growing up?")
print(response)
إنشاء الفهرس
إنشاء Embedding لكل Node
إنشاء فهرس في VectorStroreIndex
1.بالنسبة إلى VectorStoreIndex ، يتم تخزين النص embedding على العقدة في فهرس FAISS ، مما يسمح بالبحث السريع عن التشابه على العقدة ؛ 2.كما يقوم الفهرس بتخزين البيانات الفوقية على كل عقدة، مثل معرف المستند والموقع، وما إلى ذلك؛ 3.يمكن للعقدة استرجاع محتويات مستند معين أو استرجاع مستند معين.
البحث عن الفهرس
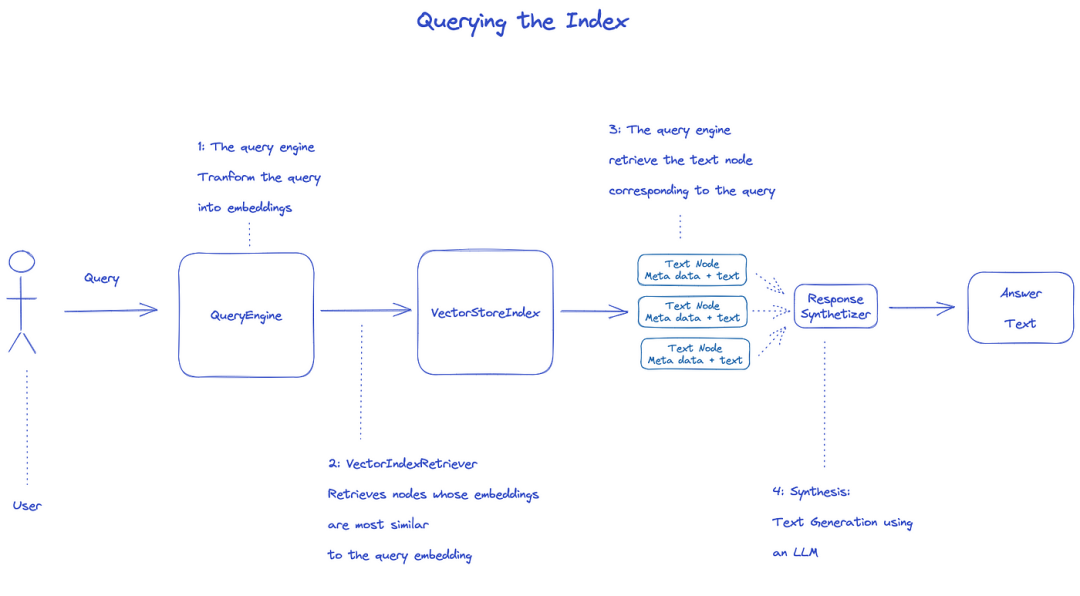
للاستعلام عن الفهرس ، سيتم استخدام QueryEngine.
1.يستحوذ Retriever على العقد ذات الصلة من فهرس الاستعلام.على سبيل المثال، يبحث VectorIndexRetriever عن العقد الأكثر تشابهًا للتembedding مع الاستعلام عن embedding؛ 2.يتم تمرير قائمة العقد التي تم استرجاعها إلى ResponseSynthesizer لإنتاج الناتج النهائي ؛ 3.بشكل افتراضي ، يتعامل ResponseSynthesizer مع كل عقدة بالتسلسل ، وتستدعي كل عقدة واجهة برمجة تطبيقات LLM مرة واحدة ؛ 4. LLM إدخال الاستفسارات ونص العقد للحصول على المخرجات النهائية؛ 5.يتم تجميع استجابات كل من هذه العقدة في سلسلة الإخراج النهائية.
from llama_index import (
VectorStoreIndex,
get_response_synthesizer,
)
from llama_index.retrievers import VectorIndexRetriever
from llama_index.query_engine import RetrieverQueryEngine
from llama_index.postprocessor import SimilarityPostprocessor
from llama_index import StorageContext, load_index_from_storage
# rebuild storage context
storage_context = StorageContext.from_defaults(persist_dir="storage")
# load index
index = load_index_from_storage(storage_context)
# configure retriever
retriever = VectorIndexRetriever(
index=index,
similarity_top_k=10,
)
# configure response synthesizer
response_synthesizer = get_response_synthesizer()
# assemble query engine
query_engine = RetrieverQueryEngine(
retriever=retriever,
response_synthesizer=response_synthesizer,
node_postprocessors=[SimilarityPostprocessor(similarity_cutoff=0.7)],
)
# query
response = query_engine.query("What did the author do growing up?")
print(response)
الوثيقة الرسمية: Understanding
** ثلاث عمليات لمعالجة البيانات **
تنظيف البيانات / خطوط أنابيب هندسة الميزات في عالم ML ، أو خطوط أنابيب ETL في إعداد البيانات التقليدية.
يتكون خط أنابيب الابتلاع هذا عادة من ثلاث مراحل رئيسية:
- تحميل البيانات
- تحويل البيانات
- فهرس وتخزين البيانات
تحميل البيانات (Ingestion)
** الهدف: ** تنسيق أنواع مختلفة من البيانات في كائنات document
.
** المدخلات: ** أنواع البيانات المختلفة
** المخرجات: ** كائن document
** ثلاث طرق **
- استخدام الفئة
SimpleDirectoryReader
: الأكثر ملاءمة Reader
فيLlamaHub
: أدوات مختلفة مكتوبة بالفعل- إنشاء
document
مباشرة
** فئة SimpleDirectoryReader
**
from llama_index import SimpleDirectoryReader
documents = SimpleDirectoryReader("./data").load_data()
دعم Markdown و PDFs و Word documents (.docx) و PowerPoint decks و images (.jpg و .png) و audio and video
**Llamahub **
- Notion (
NotionPageReader
) - Google Docs (
GoogleDocsReader
) - Slack (
SlackReader
) - Discord (
DiscordReader
) - Apify Actors (
ApifyActor
). Can crawl the web, scrape webpages, extract text content, download files including.pdf
,.jpg
,.png
,.docx
, etc.这个可以爬虫
** إنشاء الوثيقة مباشرة**
from llama_index.schema import Document
doc = Document(text="text")
تحويلات البيانات (Transformations)
** السبب: ** سهولة الوصول والاستخدام الفعال LLM
** العمليات المحددة: **
- تجزئة
الوثيقة
(تجزئة) - استخراج البيانات الفوقية (Extracting metadata)
- إدماج
** المدخلات: ** العقدة
** الناتج: ** العقدة
API بعد التغليف
طريقة from_documents
() باستخدام VectorStoreIndex
from llama_index import VectorStoreIndex
vector_index = VectorStoreIndex.from_documents(documents)
vector_index.as_query_engine()
** كيفية تخصيص المعلمات **
فكرة: استخدام ServiceContext
للتخصيص
text_splitter = SentenceSplitter(chunk_size=512, chunk_overlap=10)
service_context = ServiceContext.from_defaults(text_splitter=text_splitter)
index = VectorStoreIndex.from_documents(
documents, service_context=service_context
)
API الذرية
نموذج الاستخدام الموحد
from llama_index import Document
from llama_index.embeddings import OpenAIEmbedding
from llama_index.text_splitter import SentenceSplitter
from llama_index.extractors import TitleExtractor
from llama_index.ingestion import IngestionPipeline, IngestionCache
# 加载数据源
documents = SimpleDirectoryReader("./data").load_data()
# 创建转换数据的工作流
# create the pipeline with transformations
pipeline = IngestionPipeline(
transformations=[
SentenceSplitter(chunk_size=25, chunk_overlap=0), # 分片
TitleExtractor(), # 提取Meta信息
OpenAIEmbedding(), # Embedding
]
)
# 执行流程,生成节点
# run the pipeline
nodes = pipeline.run(documents=documents)
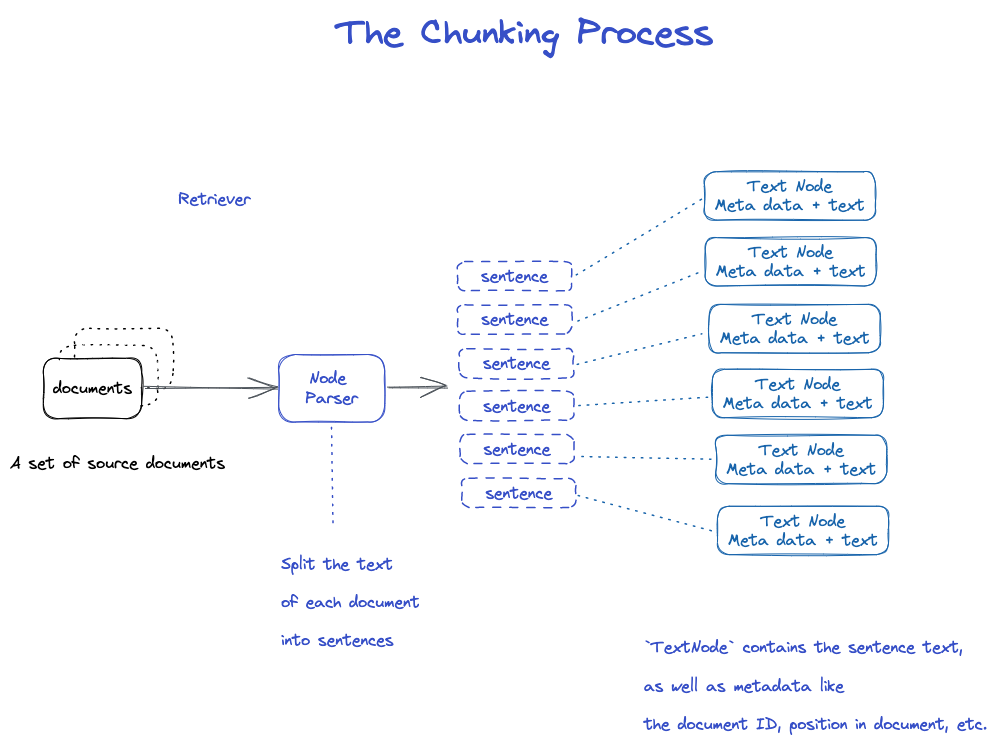
التجزئة
هناك العديد من الاستراتيجيات ، انظر وحدة Node Parser.
إضافة بيانات وصفية
يمكنك تخصيص الوثائق والعقدة وإضافة البيانات الفوقية.
إنشاء كائن Node مباشرة
from llama_index.schema import TextNode
node1 = TextNode(text="<text_chunk>", id_="<node_id>")
node2 = TextNode(text="<text_chunk>", id_="<node_id>")
index = VectorStoreIndex([node1, node2])
المرفق الأول
** تصنيف الفهارس**
- Vector Stores
- Document Stores
- Index Stores
- Key-Value Stores
- Using Graph Stores
- [متاجر الدردشة](
** الفهارس الشائعة**
- المؤشر الموجز (سابقاً مؤشر القائمة)
- Vector Store Index (الأكثر شيوعا)
- مؤشر شجرة
- مؤشر جدول الكلمات الرئيسية
Summary Index (سابقاً List Index)

** مؤشر المتجر فيكتور **

** مؤشر الأشجار **
** مؤشر جدول الكلمات الرئيسية**

ميتا
إضافة Meta
document.metadata['lang'] = lang
الترشيح
from llama_index.core.vector_stores import (
ExactMatchFilter,
MetadataFilters,
MetadataFilter,
)
filters = MetadataFilters(
filters=[
MetadataFilter(key="post_year", value="2017"),
],
)
# You pass filter as an argument. You can have any type of filter
# we saw above and then pass it to query engine.
query_engine = index.as_query_engine(service_context=service_context,
similarity_top_k=5,
filters = filters,
response_mode='tree_summarize')
response = query_engine.query("Marathon Running")
print(response)
أوضاع الاستجابة
- refine: يتم إنشاء الإجابات تلو الآخر مع context؛ استخدم قالب text_qa_template ثم قالب refine_template.
- compact: الافتراضي.على غرار refining ، إلا أنه يملأ السياق بطلب واحد.
- tree_summary
- simple_summarize
المصدر
الوثائق
a
Document
is a subclass of aNode
)
وتتضمن:
-
النص
-
metadata
-
relationships
: العلاقات مع الوثائق/العقد الأخرى
عملية استخدام الذرات
from llama_index import Document, VectorStoreIndex
# 数据源
text_list = [text1, text2, ...]
# 手动创建documents
documents = [Document(text=t) for t in text_list]
# 建立索引: 传入document,在VectorStoreIndex再转换:分片转成Node,Embedding等
index = VectorStoreIndex.from_documents(documents)
عدة طرق لإنشاء الوثائق
** تم إنشاؤه يدويا **
from llama_index import Document
text_list = [text1, text2, ...]
documents = [Document(text=t) for t in text_list]
** استخدام محمل البيانات (الموصل) **
كل منهما لديه طريقة load_data()
from llama_index import SimpleDirectoryReader
documents = SimpleDirectoryReader("./data").load_data()
** بيانات نموذجية تم إنشاؤها تلقائيا**
document = Document.example()
تخصيص Meta
from llama_index import Document
from llama_index.schema import MetadataMode
document = Document(
text="This is a super-customized document",
metadata={
"file_name": "super_secret_document.txt",
"category": "finance",
"author": "LlamaIndex",
},
excluded_llm_metadata_keys=["file_name"],
metadata_seperator="::",
metadata_template="{key}=>{value}",
text_template="Metadata: {metadata_str}\n-----\nContent: {content}",
)
print(
"The LLM sees this: \n",
document.get_content(metadata_mode=MetadataMode.LLM),
)
print()
print(
"The Embedding model sees this: \n",
document.get_content(metadata_mode=MetadataMode.EMBED),
)
الصادرات
The LLM sees this:
Metadata: category=>finance::author=>LlamaIndex
-----
Content: This is a super-customized document
The Embedding model sees this:
Metadata: file_name=>super_secret_document.txt::category=>finance::author=>LlamaIndex
-----
Content: This is a super-customized document
نمط استخدام استخراج البيانات الفوقية (غير مفهوم)
Node
الجوهر: جزء من الوثيقة
كيف تحصل على:
- استخدام فئة NodeParser لتحويل المستند إلى Node
- إنشاء يدويا
كما هو الحال مع الوثيقة:
-
النص
-
metadata
-
relationships
: العلاقات مع الوثائق/العقد الأخرى
عند التحويل من وثيقة إلى عقدة ، يتم وراث معلومات مثل البيانات الوصفية.
Node هو مواطن من الدرجة الأولى في LlamaIndex.
عملية استخدام الذرات
from llama_index.node_parser import SentenceSplitter
# load documents
...
# 手动转换:切片,转成Node
# parse nodes
parser = SentenceSplitter()
nodes = parser.get_nodes_from_documents(documents)
# build index
index = VectorStoreIndex(nodes)
** إقامة العلاقة**
from llama_index.schema import TextNode, NodeRelationship, RelatedNodeInfo
node1 = TextNode(text="text_chunk1", id_="node_id1")
node2 = TextNode(text="text_chunk2", id_="node_id2")
node3 = TextNode(text="text_chunk3", id_="node_id3")
# set relationships
node1.relationships[NodeRelationship.NEXT] = RelatedNodeInfo(
node_id=node2.node_id
)
node2.relationships[NodeRelationship.PREVIOUS] = RelatedNodeInfo(
node_id=node1.node_id
)
node2.relationships[NodeRelationship.PARENT] = RelatedNodeInfo(
node_id=node3.node_id, metadata={"key": "val"}
)
print(node2)
NodeParser
الاستخدام: تحويل مصادر البيانات إلى كائن Node
تحديداً: تقسيم مجموعة من كائنات الوثائق إلى كائنات عقدة متعددة
الإنجازات الملموسة الشائعة
NodeParser عبارة عن فئة تجريدة تم تنفيذها على وجه التحديد:
** حسب نوع الوثيقة**
- SimpleFileNodeParser
- HTMLNodeParser
- JSONNodeParser
- MarkdownNodeParser
** تقسيم النص**
- CodeSplitter
- LangchainNodeParser
- الجملةSplitter
- SentenceWindowNodeParser(غير مفهوم)
- SemanticSplitterNodeParser (لا أفهم ، يبدو متقدمًا)
- توكنتكتسبرتر
** العلاقة الأبوية**
- HierarchicalNodeParser: استخدام في AutoMergingRetriever
الاستخدامات النموذجية
** الاستخدامات الذرية**
from llama_index import Document
from llama_index.node_parser import SentenceSplitter
# 创建NodeParser
node_parser = SentenceSplitter(chunk_size=1024, chunk_overlap=20)
# 调用 get_nodes_from_documents() 方法
# show_progress 可以显示进度
nodes = node_parser.get_nodes_from_documents(
[Document.example(), Document.example()], show_progress=True
)
print(len(nodes))
print()
print(nodes[0])
الصادرات
2
Node ID: eaeb6e44-6828-4e36-b7a3-69342de4dc7c
Text: Context LLMs are a phenomenal piece of technology for knowledge
generation and reasoning. They are pre-trained on large amounts of
publicly available data. How do we best augment LLMs with our own
private data? We need a comprehensive toolkit to help perform this
data augmentation for LLMs. Proposed Solution That's where LlamaIndex
comes in. Ll...
التحولات في خط الأنابيب
from llama_index import Document
from llama_index.node_parser import SentenceSplitter
from llama_index.ingestion import IngestionPipeline
from llama_index.node_parser import TokenTextSplitter
documents = [Document.example(), Document.example()]
# 创建NodeParser
node_parser = SentenceSplitter(chunk_size=1024, chunk_overlap=20)
# 将NodeParser放到Pipeline中的transformations列表
pipeline = IngestionPipeline(transformations=[node_parser])
nodes = pipeline.run(documents=documents)
print(len(nodes))
print()
print(nodes[0])
استخدام ServiceContext
from llama_index import Document, ServiceContext, VectorStoreIndex
from llama_index.node_parser import SentenceSplitter
from llama_index.ingestion import IngestionPipeline
from llama_index.node_parser import TokenTextSplitter
documents = [Document.example(), Document.example()]
node_parser = SentenceSplitter(chunk_size=1024, chunk_overlap=20)
service_context = ServiceContext.from_defaults(text_splitter=node_parser)
index = VectorStoreIndex.from_documents(
documents, service_context=service_context, show_progress=True
)
تحويلات
إدخال: مجموعة من Node
الإخراج: مجموعة من Node
هناك طريقتان عامتان:
- `call(): مزامنة
- `acall(): غير متزامن
NodeParser و MetadataExtractor
ينتمون إلى Transformations
** نموذج الاستخدام**
from llama_index.text_splitter import SentenceSplitter
from llama_index.extractors import TitleExtractor
node_parser = SentenceSplitter(chunk_size=512)
extractor = TitleExtractor()
# use transforms directly
nodes = node_parser(documents)
# or use a transformation in async
nodes = await extractor.acall(nodes)
** بالاشتراك مع ServiceContext**
from llama_index import ServiceContext, VectorStoreIndex
from llama_index.extractors import (
TitleExtractor,
QuestionsAnsweredExtractor,
)
from llama_index.ingestion import IngestionPipeline
from llama_index.text_splitter import TokenTextSplitter
transformations = [
TokenTextSplitter(chunk_size=512, chunk_overlap=128),
TitleExtractor(nodes=5),
QuestionsAnsweredExtractor(questions=3),
]
# 创建ServiceContext,传入Transfrmation
service_context = ServiceContext.from_defaults(
transformations=[text_splitter, title_extractor, qa_extractor]
)
# 传入VectorStoreIndex的from_documents()或insert()方法
index = VectorStoreIndex.from_documents(
documents, service_context=service_context
)
الخدمةContext
حزمة من الخدمات والتكوينات المستخدمة عبر خط أنابيب LlamaIndex.
يمكن تكييفها
from llama_index import (
ServiceContext,
OpenAIEmbedding,
PromptHelper,
)
from llama_index.llms import OpenAI
from llama_index.text_splitter import SentenceSplitter
# 设置LLM
llm = OpenAI(model="text-davinci-003", temperature=0, max_tokens=256)
# 设置Embedding模型
embed_model = OpenAIEmbedding()
# 设置Chunk的大小
text_splitter = SentenceSplitter(chunk_size=1024, chunk_overlap=20)
prompt_helper = PromptHelper(
context_window=4096,
num_output=256,
chunk_overlap_ratio=0.1,
chunk_size_limit=None,
)
service_context = ServiceContext.from_defaults(
llm=llm, # 设置LLM
embed_model=embed_model, # 设置Embedding模型
text_splitter=text_splitter, # 设置Chunk的大小
prompt_helper=prompt_helper,
)
** إرسال الوظيفة البنائية ** (أكثر ملاءمة)
Kwargs for node parser:
chunk_size
- `chunk_overlap
Kwargs للمساعد السريع:
context_window
:num_output
مثلاً
service_context = ServiceContext.from_defaults(chunk_size=1000)
** التكوين العالمي**
from llama_index import set_global_service_context
set_global_service_context(service_context)
** التشكيلات المحلية**
query_engine = index.as_query_engine(service_context=service_context)
StorageContext
يحدد الواجهة الخلفية للتخزين حيث يتم تخزين المستندات والتضمينات والفهارس.
APIالمرجع
store = PGVectorStore(
connection_string=conn_string,
async_connection_string=async_conn_string,
schema_name=PGVECTOR_SCHEMA,
table_name=PGVECTOR_TABLE,
)
index = VectorStoreIndex.from_vector_store(store)
VectorStoreIndex
وظيفة البناء
index = VectorStoreIndex.from_vector_store(store)
هناك نوعان من المحرك:
- Query Engine: BaseQueryEngine
- Chat Engines: BaseChatEngine
إنشاء Engine
index.as_query_engine()# BaseQueryEngine
index.as_query_engine(streaming=True)# 流式 BaseQueryEngine
index.as_chat_engine() # BaseChatEngine; 流式不是在这里控制
الاستفسار
# Query
response = await query_engine.aquery(query) # 流式
response = await query_engine.aquery(query)
# Chat
response = await chat_engine.astream_chat(last_message_content, messages) # 流式在这里控制
response = await chat_engine.achat(last_message_content, messages)
BaseQueryEngine
استعلام
المياه
BaseChatEngine
- دردشة
- stream_chat
- شراء
- astream_chat
stream > دعم التدفق:
دعم عدم التزامن: أ بداية
أنواع الاستجابة
# Query
RESPONSE_TYPE = Union[
Response,
StreamingResponse, AsyncStreamingResponse, #流式
PydanticResponse
]
# Chat
StreamingAgentChatResponse #流式
AGENT_CHAT_RESPONSE_TYPE = Union[AgentChatResponse, StreamingAgentChatResponse] #非流式
كيفية التعامل مع ردود الفعل المتدفقة
واجهة قياسية باستخدام Python:
- StreamingResponse() -AsyncStreamingResponse
- StreamingResponse
- استفسار
@r.post("")
async def chat(
request: Request,
queryData: _QueryData,
query_engine: BaseQueryEngine = Depends(get_query_engine_stream),
):
query = queryData.query
streaming_response = await query_engine.aquery(query)
async def event_generator():
async for token in streaming_response.async_response_gen:
if await request.is_disconnected():
break
yield f"data: {token}\n\n"
return StreamingResponse(event_generator(), media_type="text/event-stream")
- الدردشة
@r.post("")
async def chat(
request: Request,
data: _ChatData,
chat_engine: BaseChatEngine = Depends(get_chat_engine),
):
last_message_content, messages = await parse_chat_data(data)
response = await chat_engine.astream_chat(last_message_content, messages)
async def event_generator():
async for token in response.async_response_gen():
if await request.is_disconnected():
break
yield token
return StreamingResponse(event_generator(), media_type="text/plain")
StreamingResponse
class AsyncStreamingResponse:
async_response_gen: TokenAsyncGen
class StreamingResponse:
response_gen: TokenGen
أوضاع الاستجابة
المراقبة
الدروس
تعليمي Deeplearn
Building and Evaluating Advanced RAG Applications:链接 Bilibili
**Joint Text to SQL and Semantic Search **
يغطي هذا الفيديو الأدوات المضمنة في LlamaIndex للجمع بين SQL والبحث الدلالي في واجهة استعلام موحدة واحدة.