LlamaIndex
Python and Typescript versions
The Python version has more complete Document but poorer ts?
introduction
Create Environment
conda create --name llamaindex python=3.9.19
conda activate llamaindex
Set up the Conda Environment in VSCode
Python: Select Interpreter
installation library
pip install llama-index pypdf sentence_transformers
Configuring OpenAI
vim ~/.bashrc
Add Environment Variables
export OPENAI_API_KEY="sk-xxxx"
verification
echo $OPENAI_API_KEY
accessibility
Configure: goproxy on the command Row
Quick Start
import os.path
from llama_index import (
VectorStoreIndex,
SimpleDirectoryReader,
StorageContext,
load_index_from_storage,
)
import logging
import sys
logging.basicConfig(stream=sys.stdout, level=logging.DEBUG)
logging.getLogger().addHandler(logging.StreamHandler(stream=sys.stdout))
# check if storage already exists
PERSIST_DIR = "./storage"
if not os.path.exists(PERSIST_DIR):
# load the documents and create the index
documents = SimpleDirectoryReader("data").load_data()
index = VectorStoreIndex.from_documents(documents)
# store it for later
index.storage_context.persist(persist_dir=PERSIST_DIR)
else:
# load the existing index
storage_context = StorageContext.from_defaults(persist_dir=PERSIST_DIR)
index = load_index_from_storage(storage_context)
# either way we can now query the index
query_engine = index.as_query_engine()
response = query_engine.query("What did the author do growing up?")
print(response)
Completions method used
/chat/completions
Parameter of Query
{
"messages": [
{
"role": "system",
"content": "You are an expert Q&A system that is trusted around the world.\nAlways answer the query using the provided context information, and not prior knowledge.\nSome rules to follow:\n1. Never directly reference the given context in your answer.\n2. Avoid statements like \"Based on the context, ...\" or \"The context information ...\" or anything along those lines."
},
{
"role": "user",
"content": "xxx"
}
],
"model": "gpt-3.5-turbo",
"stream": false,
"temperature": 0.1
}
System Prompt
You are an expert Q&A system that is trusted around the world.
Always answer the query using the provided context information, and not prior knowledge.
Some rules to follow:
1. Never directly reference the given context in your answer.
2. Avoid statements like "Based on the context, ..." or "The context information ..." or anything along those lines.
Full trusted expert question answering system. When answering questions for the hour, always use the background info provided, not previous Knowledge. Some rules to follow:
- Never directly quote given background info in your answer.
- Avoid using "based on background information,..." or "background info indicates,..." or any similar expression.
User Prompt
Context information is below.
---------------------
file_path: data/paul_graham_essay.txt
xxx
---------------------
Given the context information and not prior knowledge, answer the query.
Query: What did the author do growing up?
Answer:
application scenarios
apply field | explain |
---|---|
Q&A | most important |
Chatbots | |
Agents | advanced |
Structured Data Extraction | Useful, organize chat records, etc. |
Multi-modal |
基本原理
basic procedure
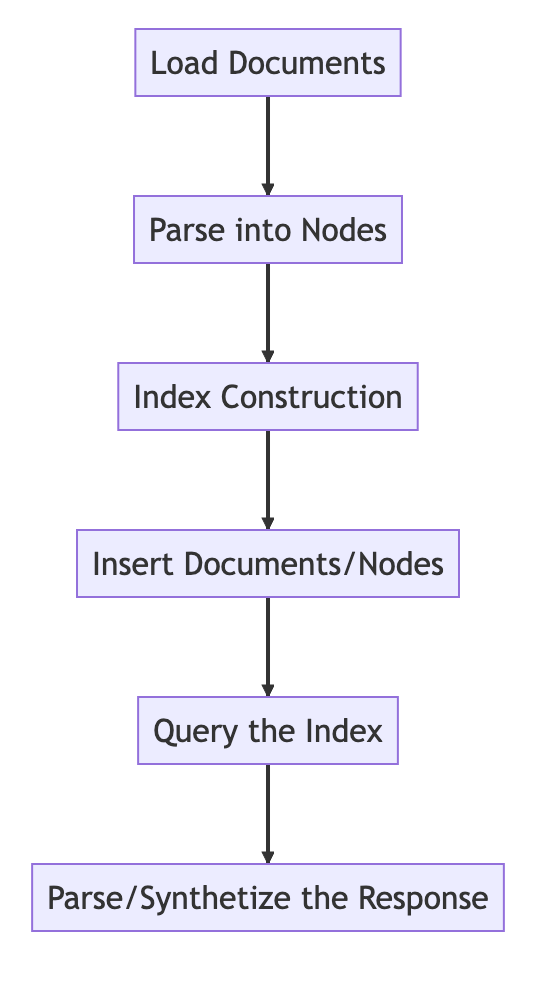
from llama_index import VectorStoreIndex, SimpleDirectoryReader
# Load in data as Document objects
documents = SimpleDirectoryReader('data').load_data()
# 切片,转成Node
# Parse Document objects into Node objects to represent chunks of data
index = VectorStoreIndex.from_documents(documents)
# Index Construction:创建索引
# Build an index over the Documents or Nodes
query_engine = index.as_query_engine()
# The response is a Response object containing the text response and source Nodes
summary = query_engine.query("What is the text about")
print("What is the data about:")
print(summary)
Chunking and Node
source Data-->documents-->Nodes
documents: Contains body text and meta info
Document ID
document is actually a subclass of Node
It's strange that a document will be cut into many documents.
Textnode: Use NodeParser to cut a document into multiple Node
Contains Document ID
Linkage Relation between Node and Node before
- NodeParser receives a list of Document objects;
- Use spaCy's sentence segmentation to segment the text of each Document into sentences;
- Each sentence is wrapped in a TextNode object, which Representation a node;
- TextNode contains sentence text, as well as Yuan Data, such as Document ID, location in the Document, etc.;
- Returns a list of TextNode objects.
Save document and index
two patterns
- Save to local disk
- Storage to vector database
Save to local disk
import os.path
from llama_index import (
VectorStoreIndex,
SimpleDirectoryReader,
StorageContext,
load_index_from_storage,
)
import sys
# check if storage already exists
PERSIST_DIR = "./storage"
if not os.path.exists(PERSIST_DIR):
# 保存数据: Load the documents and create the index
documents = SimpleDirectoryReader("data").load_data()
index = VectorStoreIndex.from_documents(documents)
# store it for later
index.storage_context.persist(persist_dir=PERSIST_DIR)
else:
# 从磁盘加载回数据: load the existing index
storage_context = StorageContext.from_defaults(persist_dir=PERSIST_DIR)
index = load_index_from_storage(storage_context)
query_engine = index.as_query_engine()
response = query_engine.query("What did the author do growing up?")
print(response)
Create Index of Matrix
Create an Embedding for each Node
Create an Index of Matrix in VectorStroreIndex
- For VectorStoreIndex, the text embeddings on the node will be Storage in the FAISS Index of Matrix, allowing for fast similarity Searching on the node;
- Index of Matrix also Storage Yuan Data on each node, such as document ID, location, etc.;
- Nodes can retrieve the content of a Document or a specific Document.
Query Index of Matrix
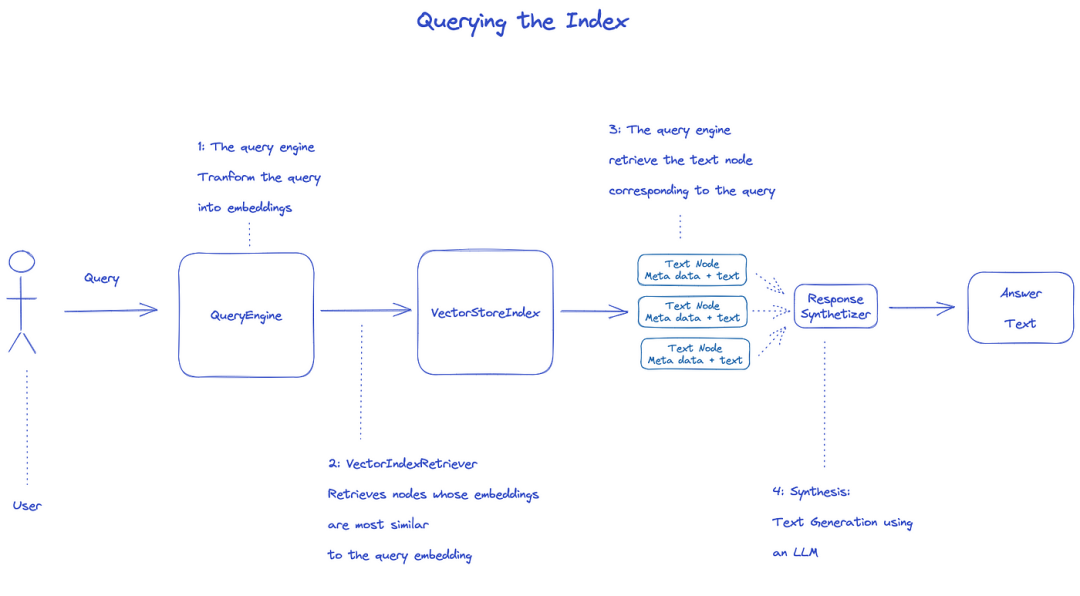
Query the Index of Matrix using QueryEngine.
- Retriever retrieves the correlation nodes from the Index of Matrix of the Query. For example, VectorIndexRetriever retrieves the node whose embedding is most similar to the Query embedding;
- The retrieved node list is passed to the ResponseSynthesizer to generate the final output;
- By default, the ResponseSynthesizer treament each node in order, and each node callsLLM API once;
- LLM Input Query and node text to get the final output;
- These per-node responses are Agglomerative into the final output string.
from llama_index import (
VectorStoreIndex,
get_response_synthesizer,
)
from llama_index.retrievers import VectorIndexRetriever
from llama_index.query_engine import RetrieverQueryEngine
from llama_index.postprocessor import SimilarityPostprocessor
from llama_index import StorageContext, load_index_from_storage
# rebuild storage context
storage_context = StorageContext.from_defaults(persist_dir="storage")
# load index
index = load_index_from_storage(storage_context)
# configure retriever
retriever = VectorIndexRetriever(
index=index,
similarity_top_k=10,
)
# configure response synthesizer
response_synthesizer = get_response_synthesizer()
# assemble query engine
query_engine = RetrieverQueryEngine(
retriever=retriever,
response_synthesizer=response_synthesizer,
node_postprocessors=[SimilarityPostprocessor(similarity_cutoff=0.7)],
)
# query
response = query_engine.query("What did the author do growing up?")
print(response)
Official Document: Understanding
Three processes of data handling
data cleaning/feature engineering pipelines in the ML world, or ETL pipelines in the traditional data setting.
This ingestion pipeline typically consists of three main stages:
- Load the data
- Transform the data
- Index and store the data
Loading Data (Ingestion)
**Objective:**Format Various types of Data intodocument
objects.
**Input:**Various types of Data
output:document
object
3 ways
- Using
SimpleDirectoryReader
classes: the most convenient Reader
inLlamaHub
: Various tools that have been written- Create
document
directly
SimpleDirectoryReader
classes
from llama_index import SimpleDirectoryReader
documents = SimpleDirectoryReader("./data").load_data()
Supports Markdown, PDFs, Word documents(.docx), PowerPoint decks, images(.jpg, .png), audio and video
Llamahub
- Notion (
NotionPageReader
) - Google Docs (
GoogleDocsReader
) - Slack (
SlackReader
) - Discord (
DiscordReader
) - Apify Actors (
ApifyActor
). Can crawl the web, scrape webpages, extract text content, download files including.pdf
,.jpg
,.png
,.docx
, etc. This can crawl
Create document directly
from llama_index.schema import Document
doc = Document(text="text")
Data transformation (Transformations)
**Reason:**Convenient search and efficient use ofLLM
Specific Operation:
- Fragmentation (chunking) of
document
- Yuan Data extraction
- Embedding
Input:Node
output:Node
API after package
Use thefrom_documents
() methodVectorStoreIndex
from llama_index import VectorStoreIndex
vector_index = VectorStoreIndex.from_documents(documents)
vector_index.as_query_engine()
How to customize Parameter
Idea: UseServiceContext
to customize
text_splitter = SentenceSplitter(chunk_size=512, chunk_overlap=10)
service_context = ServiceContext.from_defaults(text_splitter=text_splitter)
index = VectorStoreIndex.from_documents(
documents, service_context=service_context
)
the atomic API
Standard Usage Pattern
from llama_index import Document
from llama_index.embeddings import OpenAIEmbedding
from llama_index.text_splitter import SentenceSplitter
from llama_index.extractors import TitleExtractor
from llama_index.ingestion import IngestionPipeline, IngestionCache
# 加载数据源
documents = SimpleDirectoryReader("./data").load_data()
# 创建转换数据的工作流
# create the pipeline with transformations
pipeline = IngestionPipeline(
transformations=[
SentenceSplitter(chunk_size=25, chunk_overlap=0), # 分片
TitleExtractor(), # 提取Meta信息
OpenAIEmbedding(), # Embedding
]
)
# 执行流程,生成节点
# run the pipeline
nodes = pipeline.run(documents=documents)
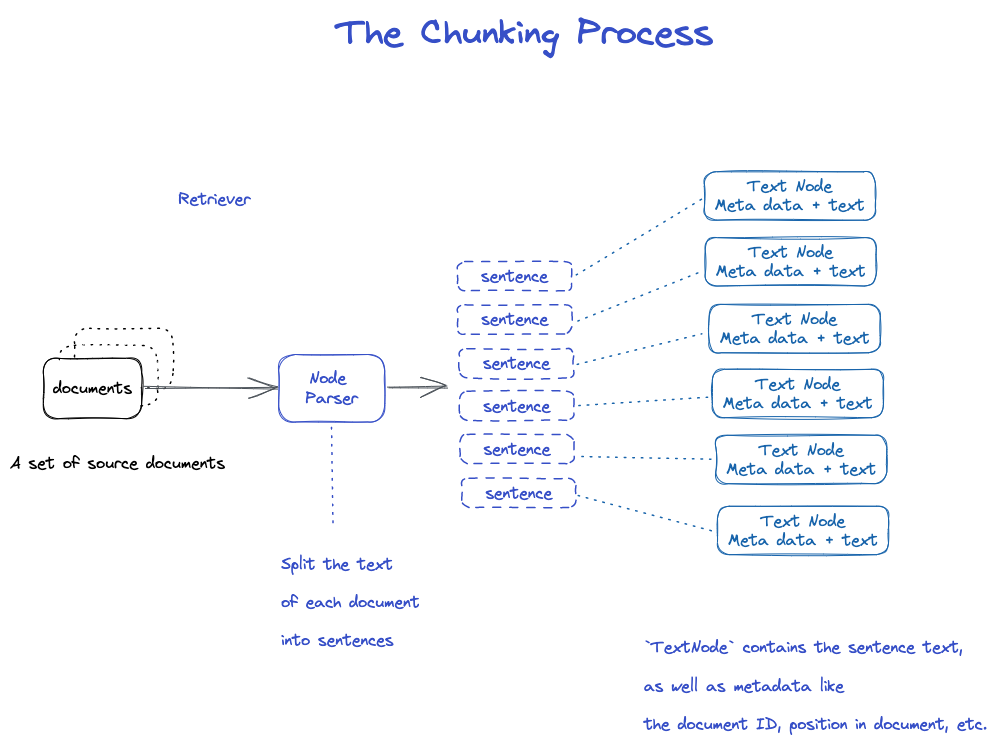
sharding
There are many Policy, see the Node Parser module for details.
Add Yuan Data
You can customize document and Node and add Yuan Data.
Create Node objects directly
from llama_index.schema import TextNode
node1 = TextNode(text="<text_chunk>", id_="<node_id>")
node2 = TextNode(text="<text_chunk>", id_="<node_id>")
index = VectorStoreIndex([node1, node2])
Index of Matrix
Index of Matrix classification
- Vector Stores
- Document Stores
- Index Stores
- Key-Value Stores
- Using Graph Stores
- [Chat Stores](
Common Index of Matrix
- Summary Index (formerly List Index)
- Vector Store Index (most common)
- Tree Index
- Keyword Table Index
Summary Index (formerly List Index)

Vector Store Index

Tree Index
Keyword Table Index

Meta
Add meta
document.metadata['lang'] = lang
filtration
from llama_index.core.vector_stores import (
ExactMatchFilter,
MetadataFilters,
MetadataFilter,
)
filters = MetadataFilters(
filters=[
MetadataFilter(key="post_year", value="2017"),
],
)
# You pass filter as an argument. You can have any type of filter
# we saw above and then pass it to query engine.
query_engine = index.as_query_engine(service_context=service_context,
similarity_top_k=5,
filters = filters,
response_mode='tree_summarize')
response = query_engine.query("Marathon Running")
print(response)
Response Modes
- refine: Generate answers one by one according to the context; use the text_qa_template template first, then use the refine_template template.
- compact: default. Similar to refine, but the context is stuffed into a request.
- tree_summarize
- simple_summarize
sound code
Document
a
Document
is a subclass of aNode
)
Contains:
-
text
-
metadata
-
relationships
: Relation with other Documents/Nodes
Atom usage process
from llama_index import Document, VectorStoreIndex
# 数据源
text_list = [text1, text2, ...]
# 手动创建documents
documents = [Document(text=t) for t in text_list]
# 建立索引: 传入document,在VectorStoreIndex再转换:分片转成Node,Embedding等
index = VectorStoreIndex.from_documents(documents)
Several ways to create a document
manually create
from llama_index import Document
text_list = [text1, text2, ...]
documents = [Document(text=t) for t in text_list]
Use the Data loader (connector)
They all have a method load_Data()
from llama_index import SimpleDirectoryReader
documents = SimpleDirectoryReader("./data").load_data()
Automatically generated example Data
document = Document.example()
Custom Meta
from llama_index import Document
from llama_index.schema import MetadataMode
document = Document(
text="This is a super-customized document",
metadata={
"file_name": "super_secret_document.txt",
"category": "finance",
"author": "LlamaIndex",
},
excluded_llm_metadata_keys=["file_name"],
metadata_seperator="::",
metadata_template="{key}=>{value}",
text_template="Metadata: {metadata_str}\n-----\nContent: {content}",
)
print(
"The LLM sees this: \n",
document.get_content(metadata_mode=MetadataMode.LLM),
)
print()
print(
"The Embedding model sees this: \n",
document.get_content(metadata_mode=MetadataMode.EMBED),
)
output
The LLM sees this:
Metadata: category=>finance::author=>LlamaIndex
-----
Content: This is a super-customized document
The Embedding model sees this:
Metadata: file_name=>super_secret_document.txt::category=>finance::author=>LlamaIndex
-----
Content: This is a super-customized document
Metadata Extraction Usage Pattern (don't understand)
Node
Essence: Fragmentation of document
How to get:
- Use the NodeParser classes to convert a document to a Node
- manually create
Like the document, there are:
-
text
-
metadata
-
relationships
: Relation with other Documents/Nodes
When converting from document to Node by the hour, info such as metadata will be inherited.
Node is a first-class citizen in LlamaIndex.
Atom usage process
from llama_index.node_parser import SentenceSplitter
# load documents
...
# 手动转换:切片,转成Node
# parse nodes
parser = SentenceSplitter()
nodes = parser.get_nodes_from_documents(documents)
# build index
index = VectorStoreIndex(nodes)
Set Relation
from llama_index.schema import TextNode, NodeRelationship, RelatedNodeInfo
node1 = TextNode(text="text_chunk1", id_="node_id1")
node2 = TextNode(text="text_chunk2", id_="node_id2")
node3 = TextNode(text="text_chunk3", id_="node_id3")
# set relationships
node1.relationships[NodeRelationship.NEXT] = RelatedNodeInfo(
node_id=node2.node_id
)
node2.relationships[NodeRelationship.PREVIOUS] = RelatedNodeInfo(
node_id=node1.node_id
)
node2.relationships[NodeRelationship.PARENT] = RelatedNodeInfo(
node_id=node3.node_id, metadata={"key": "val"}
)
print(node2)
NodeParser
Usage: Convert Datasource into Node objects
Specifically: fragment a group of document objects into multiple Node objects
Common specific implementations
NodeParser is an abstract classes, and its specific implementations include:
by file type
- SimpleFileNodeParser
- HTMLNodeParser
- JSONNodeParser
- MarkdownNodeParser
text segmentation
- CodeSplitter
- LangchainNodeParser
- SentenceSplitter
- SentenceWindowNodeParser (don't understand)
- SemanticSplitterNodeParser (I don't understand, it feels more advanced)
- TokenTextSplitter
Father-son Relation
- HierarchicalNodeParser: used in AutoMergingRetriever
typical usage
atomic use
from llama_index import Document
from llama_index.node_parser import SentenceSplitter
# 创建NodeParser
node_parser = SentenceSplitter(chunk_size=1024, chunk_overlap=20)
# 调用 get_nodes_from_documents() 方法
# show_progress 可以显示进度
nodes = node_parser.get_nodes_from_documents(
[Document.example(), Document.example()], show_progress=True
)
print(len(nodes))
print()
print(nodes[0])
output
2
Node ID: eaeb6e44-6828-4e36-b7a3-69342de4dc7c
Text: Context LLMs are a phenomenal piece of technology for knowledge
generation and reasoning. They are pre-trained on large amounts of
publicly available data. How do we best augment LLMs with our own
private data? We need a comprehensive toolkit to help perform this
data augmentation for LLMs. Proposed Solution That's where LlamaIndex
comes in. Ll...
Transformations in Pipline
from llama_index import Document
from llama_index.node_parser import SentenceSplitter
from llama_index.ingestion import IngestionPipeline
from llama_index.node_parser import TokenTextSplitter
documents = [Document.example(), Document.example()]
# 创建NodeParser
node_parser = SentenceSplitter(chunk_size=1024, chunk_overlap=20)
# 将NodeParser放到Pipeline中的transformations列表
pipeline = IngestionPipeline(transformations=[node_parser])
nodes = pipeline.run(documents=documents)
print(len(nodes))
print()
print(nodes[0])
Using ServiceContext
from llama_index import Document, ServiceContext, VectorStoreIndex
from llama_index.node_parser import SentenceSplitter
from llama_index.ingestion import IngestionPipeline
from llama_index.node_parser import TokenTextSplitter
documents = [Document.example(), Document.example()]
node_parser = SentenceSplitter(chunk_size=1024, chunk_overlap=20)
service_context = ServiceContext.from_defaults(text_splitter=node_parser)
index = VectorStoreIndex.from_documents(
documents, service_context=service_context, show_progress=True
)
Transformations
Input: a set of Node
output: a set of Node
There are two public methods:
__call__()
: Synchronizationacall()
: Asynchronous
NodeParser andMetadataExtractor
belong to Transformations
Usage Pattern
from llama_index.text_splitter import SentenceSplitter
from llama_index.extractors import TitleExtractor
node_parser = SentenceSplitter(chunk_size=512)
extractor = TitleExtractor()
# use transforms directly
nodes = node_parser(documents)
# or use a transformation in async
nodes = await extractor.acall(nodes)
Used in combination with ServiceContext
from llama_index import ServiceContext, VectorStoreIndex
from llama_index.extractors import (
TitleExtractor,
QuestionsAnsweredExtractor,
)
from llama_index.ingestion import IngestionPipeline
from llama_index.text_splitter import TokenTextSplitter
transformations = [
TokenTextSplitter(chunk_size=512, chunk_overlap=128),
TitleExtractor(nodes=5),
QuestionsAnsweredExtractor(questions=3),
]
# 创建ServiceContext,传入Transfrmation
service_context = ServiceContext.from_defaults(
transformations=[text_splitter, title_extractor, qa_extractor]
)
# 传入VectorStoreIndex的from_documents()或insert()方法
index = VectorStoreIndex.from_documents(
documents, service_context=service_context
)
ServiceContext
a bundle of services and configurations used across a LlamaIndex pipeline.
may be configured
from llama_index import (
ServiceContext,
OpenAIEmbedding,
PromptHelper,
)
from llama_index.llms import OpenAI
from llama_index.text_splitter import SentenceSplitter
# 设置LLM
llm = OpenAI(model="text-davinci-003", temperature=0, max_tokens=256)
# 设置Embedding模型
embed_model = OpenAIEmbedding()
# 设置Chunk的大小
text_splitter = SentenceSplitter(chunk_size=1024, chunk_overlap=20)
prompt_helper = PromptHelper(
context_window=4096,
num_output=256,
chunk_overlap_ratio=0.1,
chunk_size_limit=None,
)
service_context = ServiceContext.from_defaults(
llm=llm, # 设置LLM
embed_model=embed_model, # 设置Embedding模型
text_splitter=text_splitter, # 设置Chunk的大小
prompt_helper=prompt_helper,
)
Construct Function to Pass Parameters(More Convenient)
Kwargs for node parser:
chunk_size
- `chunk_overlap
Kwargs for prompt helper:
context_window
:num_output
such as
service_context = ServiceContext.from_defaults(chunk_size=1000)
global configuration
from llama_index import set_global_service_context
set_global_service_context(service_context)
local configuration
query_engine = index.as_query_engine(service_context=service_context)
StorageContext
defines the storage backend for where the documents, embeddings, and indexes are stored.
API Reference
store = PGVectorStore(
connection_string=conn_string,
async_connection_string=async_conn_string,
schema_name=PGVECTOR_SCHEMA,
table_name=PGVECTOR_TABLE,
)
index = VectorStoreIndex.from_vector_store(store)
VectorStoreIndex
Constructor Function
index = VectorStoreIndex.from_vector_store(store)
There are two types of Engines:
- Query Engine: BaseQueryEngine
- Chat Engines: BaseChatEngine
Create Engine
index.as_query_engine()# BaseQueryEngine
index.as_query_engine(streaming=True)# 流式 BaseQueryEngine
index.as_chat_engine() # BaseChatEngine; 流式不是在这里控制
Query
# Query
response = await query_engine.aquery(query) # 流式
response = await query_engine.aquery(query)
# Chat
response = await chat_engine.astream_chat(last_message_content, messages) # 流式在这里控制
response = await chat_engine.achat(last_message_content, messages)
BaseQueryEngine
query
aquery
BaseChatEngine
- chat
- stream_chat
- achat
- astream_chat
Support streaming: stream
Support for Asynchronous: beginning with a
the type of response
# Query
RESPONSE_TYPE = Union[
Response,
StreamingResponse, AsyncStreamingResponse, #流式
PydanticResponse
]
# Chat
StreamingAgentChatResponse #流式
AGENT_CHAT_RESPONSE_TYPE = Union[AgentChatResponse, StreamingAgentChatResponse] #非流式
How to handle treament responses
Use Python's standard interface:
- StreamingResponse()
- AsyncStreamingResponse
- StreamingResponse
- Query
@r.post("")
async def chat(
request: Request,
queryData: _QueryData,
query_engine: BaseQueryEngine = Depends(get_query_engine_stream),
):
query = queryData.query
streaming_response = await query_engine.aquery(query)
async def event_generator():
async for token in streaming_response.async_response_gen:
if await request.is_disconnected():
break
yield f"data: {token}\n\n"
return StreamingResponse(event_generator(), media_type="text/event-stream")
- Chat
@r.post("")
async def chat(
request: Request,
data: _ChatData,
chat_engine: BaseChatEngine = Depends(get_chat_engine),
):
last_message_content, messages = await parse_chat_data(data)
response = await chat_engine.astream_chat(last_message_content, messages)
async def event_generator():
async for token in response.async_response_gen():
if await request.is_disconnected():
break
yield token
return StreamingResponse(event_generator(), media_type="text/plain")
StreamingResponse
class AsyncStreamingResponse:
async_response_gen: TokenAsyncGen
class StreamingResponse:
response_gen: TokenGen
Response Modes
monitoring
course
Deelearn tutorial
Building and Evaluating Advanced RAG Applications:Links toBilibili
Joint Text to SQL and Semantic Search
This video covers the tools built into LlamaIndex for combining SQL and semantic search into a single unified query interface.